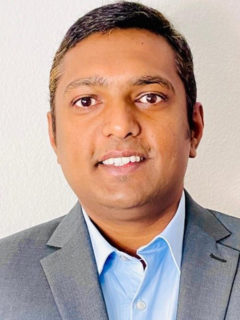
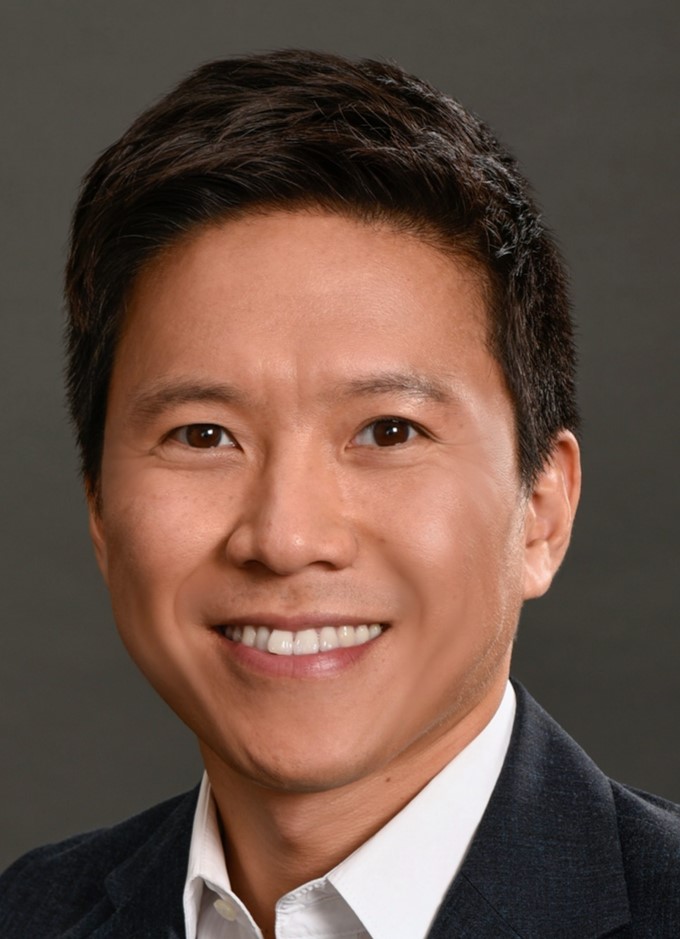
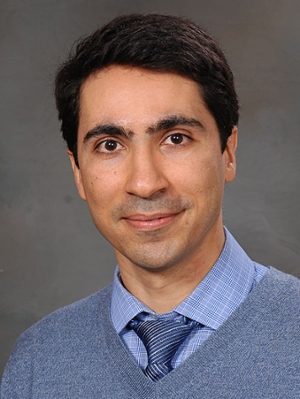
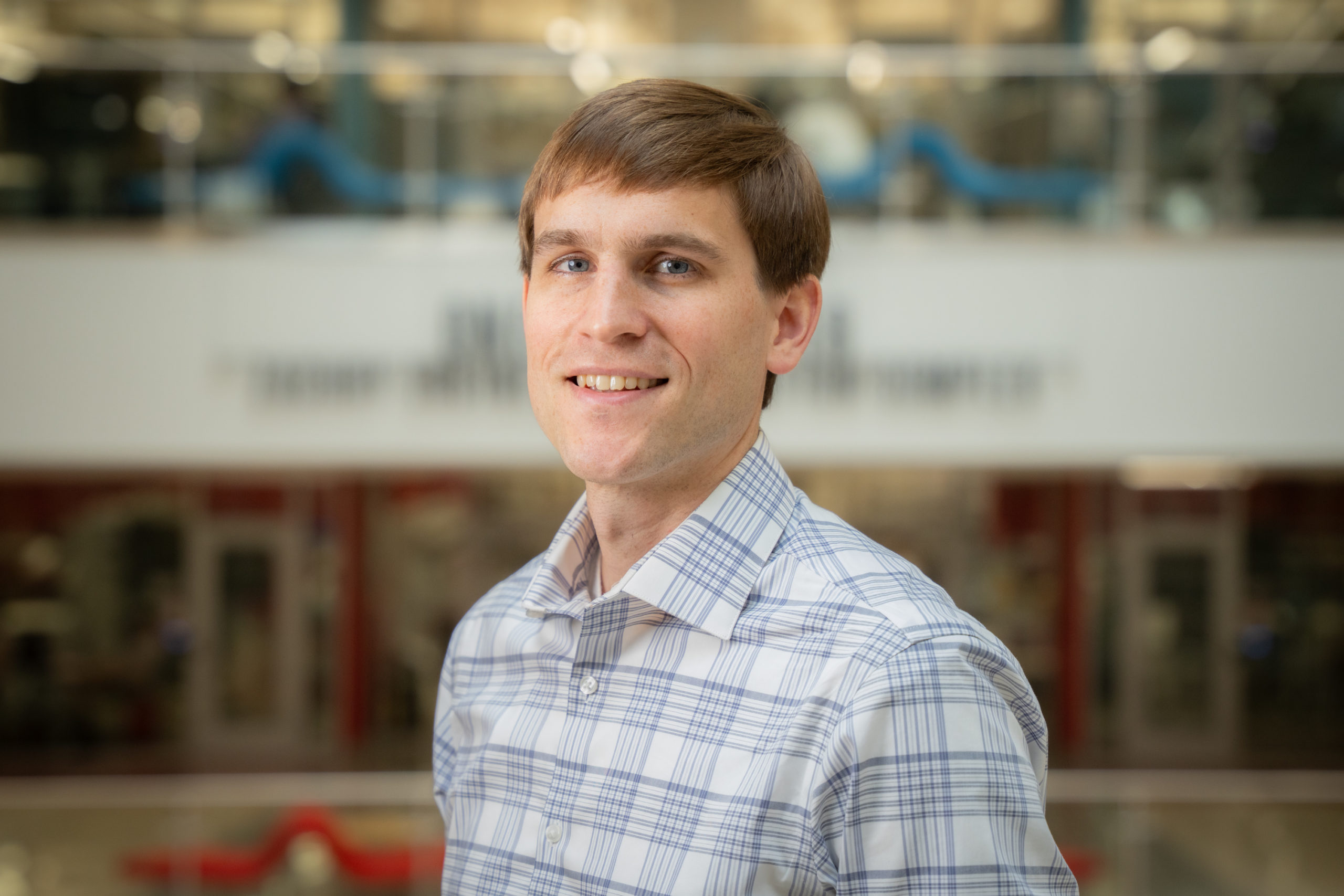
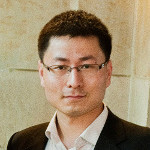
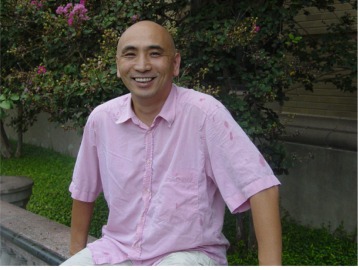
The Texas A&M Institute of Data Science (TAMIDS) has announced six faculty awards that will support the creation of new courses and materials to expand AI, Data Science, and Machine Learning literacy at Texas A&M. The TAMIDS Data Science Course Development Grant Program is offered in association with the Texas A&M Center for Teaching Excellence and offers funding to support course development across the University. This year’s recipients proposed topics that span from smart agriculture to architecture to psychology. The overwhelming number of proposals we received and the potential of these chosen courses showcase this community’s passion for expanding AI and Data Science in education. Below is a list of the 2025 awardees, their proposal titles, and the course description. Thank you to all who submitted, and our wonderful review committee for making this program such a success!
Dr. Karun Kaniyattam, Department of Animal Science: Decision Science for Sustainable Livestock Systems
Decision Science for Sustainable Livestock Systems prepares students to contribute to sustainable livestock systems by teaching data analytics and AI techniques across seven modules. It covers topics from basic data handling to advanced machine learning, deep learning, and agent-based modeling, with practical case studies throughout. Undergraduates will explore the importance of AI in their careers with minimal programming required, while graduate students will focus on applying these techniques to research using real-world data.
Dr. Namgyun Kim, Department of Construction Science: AI-Enabled Human Factors Research in Construction
AI-Enabled Human Factors Research in Construction combines construction science and artificial intelligence to address the urgent need for improved worker safety and health. Students will learn to use wearable sensors, process raw data, and apply machine learning, generative AI, and statistics to real-world construction safety challenges within a curriculum tailored to the unique challenges of construction safety research.
Dr. Arash Noshadravan, Department of Civil & Environmental Engineering: Uncertainty-Aware Machine Learning for Resilient Civil and Energy Infrastructure
Uncertainty-Aware Machine Learning for Resilient Civil and Energy Infrastructure teaches students how to develop robust machine learning models capable of delivering reliable predictions with quantifiable uncertainty, with a focus on their application to assess and enhance the performance, safety, and resilience of civil and energy infrastructure systems. Students will gain a strong foundation in uncertainty quantification, learn to integrate it with advanced ML techniques, and develop hands-on technical skills to apply these tools to solve real-world challenges in engineering systems through a multidisciplinary, data-driven approach.
Dr. Nate Veldt, Department of Computer Science and Engineering: Computational Methods for Network Science
Computational Methods for Network Science will introduce the core computational methods and optimization techniques that power modern data science and machine learning, especially for network-structured data analysis. The course will give students a solid understanding of how these tools work and why they are effective. By the end of the course, students will be able to formulate data science and network science tasks as optimization problems, choose and apply appropriate algorithms for different types of problems, and understand the strengths and limitations of various computational methods.
Dr. Jinbo Wang, Department of Oceanography: Ocean Sciences from Space
Ocean Sciences from Space targets upper-level undergraduates and graduate students interested in marine science, big data, and space technology. It introduces foundational oceanography, key remote sensing techniques, and their applications in physical oceanography and dynamics, cloud-based data access, and machine learning (ML) for data analysis. Students will be able to explore the history of satellite oceanography, gain analytical skills for satellite data, and learn the role of machine learning in interpreting remote sensing data.
Dr. Takashi Yamauchi, Department of Psychological & Brain Sciences: Artificial Intelligence (AI) and Machine Learning (ML) for Psychology
Artificial Intelligence (AI) and Machine Learning (ML) for Psychology provides undergraduate and graduate students in psychology and neuroscience with a foundational understanding of artificial intelligence and machine learning and their applications in psychological research. Students will explore the historical development and basic principles of AI and ML, as well as their risks, limitations, and ethical concerns. The course emphasizes hands-on skill development, including Python programming and the implementation of AI/ML models on large archival data.